Haydn Belfield [1] is a Research Associate and Academic Project Manager at the University of Cambridge’s Centre for the Study of Existential Risk. He is also an Associate Fellow at the Leverhulme Centre for the Future of Intelligence. He works on the international security applications of emerging technologies, especially artificial intelligence. He has a background in policy and politics, including as a Senior Parliamentary Researcher to a British Shadow Cabinet Minister, as a Policy Associate to the University of Oxford’s Global Priorities Project, and a degree in Philosophy, Politics and Economics from Oriel College, University of Oxford.
[1]hb492@cam.ac.uk
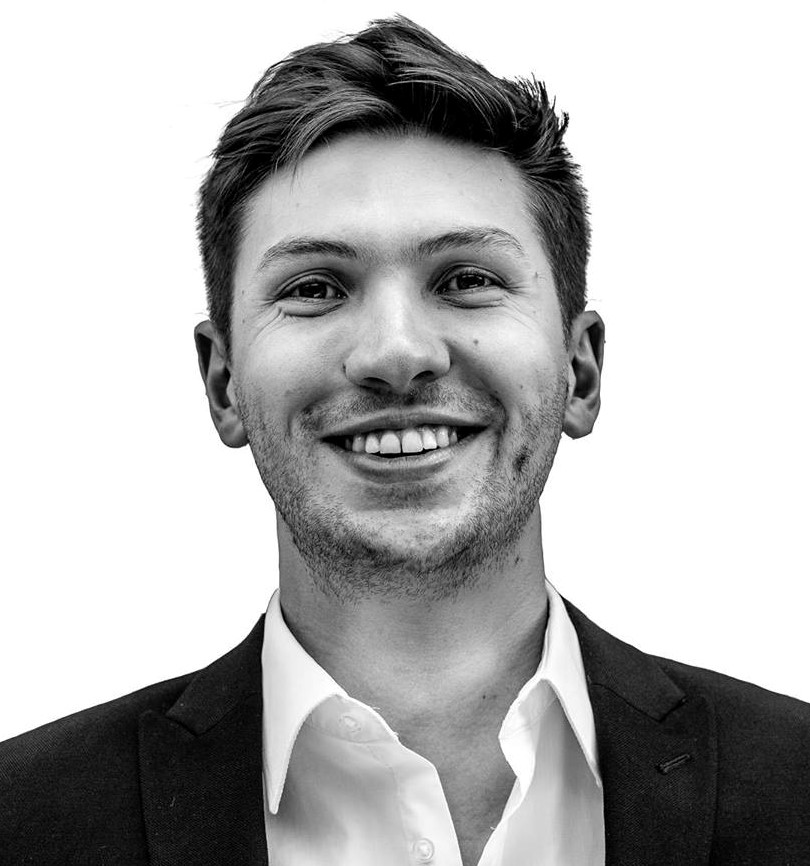
Artificial intelligence (AI) is beginning to change our world – for better and for worse. Like any other powerful and useful technology, it can be used both to help and to harm. We explored this in a major Febuary 2018 report The Malicious Use of Artificial Intelligence: Forecasting, Prevention, and Mitigation.[1] We co-authored this report with 26 international experts from academia and industry to assess how criminals, terrorists and rogue states could maliciously use AI over the next five years, and how these misuses might be prevented and mitigated. In this piece I will cover recent advances in artificial intelligence, some of the new threats these pose, and what can be done about it.
In this piece I will cover recent advances in artificial intelligence, some of the new threats these pose, and what can be done about it.
AI, according to Nilsson, “is that activity devoted to making machines intelligent, and intelligence is that quality that enables an entity to function appropriately and with foresight in its environment”.[2] It has been a field of study from at least Alan Turing in the 1940s, and perhaps from Ada Lovelace in the 1840s. Most of the interest in recent years has come from the subfield of ‘machine learning’, in which instead of writing lots of explicit rules, one trains a system (or ‘model’) on data and the system ‘learns’ to carry out a particular task. Over the last few years there has been a notable increase in the capabilities of AI systems, and an increase in access to those capabilities.
The increase in AI capabilities is often dated from 2012’s seminal Alexnet paper.[3] This system achieved a big jump in capabilities on an image recognition task. This task has now been so comprehensively beaten that it has become a benchmark for new systems – “this method achieves state-of-the-art in less time, or at a lower cost”. Advances in natural language processing (NLP) have led to systems capable of advanced translation, comprehension and analysis of text and audio – and indeed the creation of synthetic text (OpenAI’s GPT-2) and audio (Google’s Duplex). Generative Adversarial Networks (GANs) are capable of creating incredibly convincing synthetic images and videos. The UK company DeepMind achieved fame within the AI field with their systems capable of beating Atari games from the 1980s such as Pong. But they broke into the popular imagination with their AlphaGo systems defeat of Lee Sedol at Go. AlphaGo Zero, the successor program, was also superhuman at Chess and Shogi. AI systems have continued to match or surpass human performance at more games, and more complicated games: fast-paced, complex, ‘real-time strategy’ games such as DOTA II and Starcraft II.
This increase has been driven by key conceptual breakthroughs, the application of lots of money and talented people, and an increase in computing power (or ‘compute’). For example, training AlphaGo Zero used 300,000 times as much compute as AlexNet.[4]
Access to AI systems has also increased. Most ML papers are freely, openly published by default on the online depository arXiv. Often the code or trained AI system can be freely downloaded from open source software libraries like GitHub or TensorFlow, which also tend to standardise programming methods. People new to the field can get up to speed through online courses such as Coursera, or the many tutorials available on YouTube. Instead of training their systems on their own computers, people can easily and cheaply train them on cloud computing providers such as Amazon Web Services or Microsoft Azure. Indeed the computer chips best suited to machine learning (GPUs and TPUs) are so expensive that it normally makes more sense to use a cloud provider, and only rent the time one needs. Overall then, it has become much easier, quicker and cheaper for someone to get up to speed, and create a working system of their own.
These two processes have had many benefits: new scientific advances, better and cheaper goods and services, and access to advanced capabilities from around the world. However they have also uncovered new vulnerabilities. One is the discovery of ‘adversarial examples’ – adjustments to input data so minor to be imperceptible to humans, but that cause a system to misclassify an input. For example, misclassifying a picture of a stop sign as a 45 mph speed limit sign.
These vulnerabilities has prompted some important work on ‘AI safety’, that is, reducing the risk of accidents involving AI systems in the short-term [6,7] and long-term.[8] Our report focussed, however, on AI security: reducing the risk of malicious use of AI by humans. We looked at the short-term: systems either currently or soon to be in use in the next five years.
AI is a ‘dual-use’ technology – it can be used for good or ill. Indeed it has been described as an ‘omni-use’ technology as it can be used in so many settings. Across many different areas however, common threat factors emerge. Existing threats are expanding, as automation allows a greater scale of attacks. The skill transfer and diffusion of capabilities described above will allow a wider range of people to carry out attacks that currently the preserve of experts. Novel threats are emerging, using the superhuman performance and speed of AI systems, or attacking the unique vulnerabilities of AI systems. The character of threats is being altered as attacks become more customised to particular targets, and the distance between target and attacker makes attacks harder to attribute.
These common factors will affect security in different ways – we split them into three domains.
In ‘digital security’, for example, current ‘spear phishing’ emails are tailor-made for a particular victim. An attacker trawls through all the information they can find on a target, and drafts a message aimed at that target. This process could be automated through the use of AI. An AI could trawl social media profiles for information, and draft tailored synthetic text. Attacks shift from being handcrafted to mass-produced.
In ‘physical security’, for example, civilian drones are likely to be repurposed for attacks. The Venezuelan regime claims to have been targeted by a drone assassination. Even if, as is most likely, this is propaganda, it gives an indication of threats to come. The failure of British police for several days to deal with a remote-controlled drone over Gatwick airport does not bode well.
In ‘political security’ or ‘epistemic security’, the concern is both that in repressive societies governments are using advanced data analytics to better surveil their populations and profile dissidents; and that in democratic societies polities are being polarised and manipulated through synthetic media and targeted political advertising.
We made several recommendations for policy-makers, technical researchers and engineers, company executives, and wide range of other stakeholders. Since we published the report, it has received global media coverage and was welcomed by experts in different domains, such as AI policy, cybersecurity, and machine learning. We have subsequently consulted several governments, companies and civil society groups on the recommendations of this report. It was featured in the House of Lords Select Committee on AI’s Report. We have run a workshop series on Epistemic Security with the Alan Turing Institute. The topic has received a great deal of coverage, due in part to the Cambridge Analytica scandal and Zuckerberg’s testimony to Congress. The Association for Computing Machinery (ACM) has called for impact assessment in the peer review process. OpenAI decided not to publish the full details of their GPT-2 system due to concerns about synthetic media. On physical security, the topic of Lethal Autonomous Weapons Systems has burst into the mainstream with the controversy around Google’s Project MAVEN.
Despite these promising developments, there is a lot still more to be done to research and develop policy around the malicious use of artificial intelligence, so that we can reap the benefits and avoid the misuse of this transformative technology. The technology is developing rapidly, and malicious actors are quickly adapting it to malicious ends. There is no time to wait.
Read more:
[1] | Brundage, M., Avin, S., et al. (2018). The Malicious Use of Artificial Intelligence: Forecasting, Prevention, and Mitigation, arXiv:1802.07228. |
[2] | Nilsson, N. J. (2009). The quest for artificial intelligence. Cambridge University Press. |
[3] | Krizhevsky, A., Sutskever, I., Hinton, G. E. (2012). Imagenet classification with deep convolutional neural networks. Advances in neural information processing systems (pp. 1097-1105). |
[4] | Amodei, D. Hernandez, D. (2018). AI and Compute. OpenAI: https://blog.openai.com/ai-and-compute/. |
[5] | Karpathy, A. (2015) Breaking Convnets. http://karpathy.github.io/2015/03/30/breaking-convnets. |
[6] | Amodei, D., Olah, C. et al. (2016) Concrete Problems in AI Safety. |
[7] | Leike, J. et al. (2017) AI Safety Gridworlds. DeepMind. |
[8] | Bostrom, N. (2014) Superintelligence. Oxford University Press. |
[9] | House of Lords Select Committee on Artificial Intelligence (2018). Report of Session AI in the UK: ready, willing and able? 2017–19 HL Paper 100. |